The landing page
is not found
You may have misstyped the address
or the page may have moved.
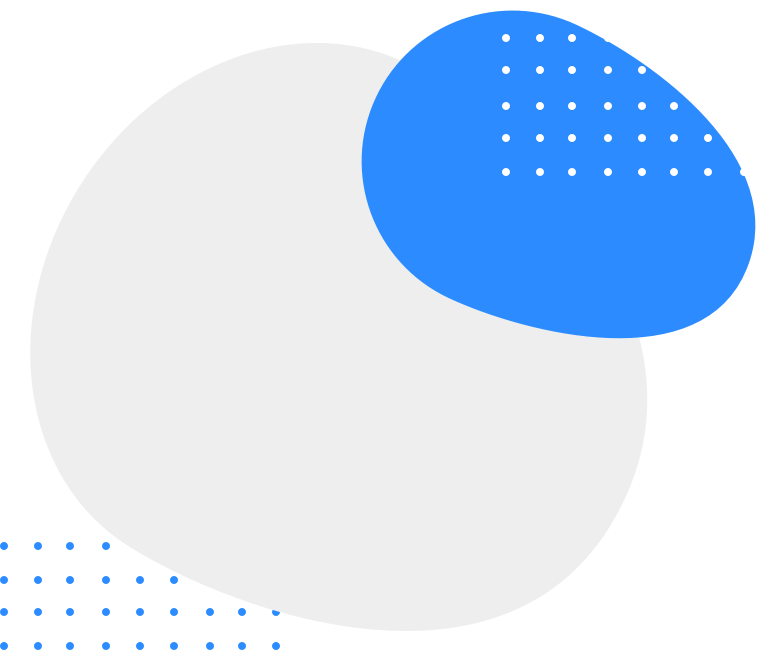
You may have misstyped the address
or the page may have moved.